LLMを使って開発を進めていると、モデルの応答の形式が安定しなくて困ったことがあると思います。
型を指定するプロンプトを書いても、必ずしもその通りに応答してくれるとはかぎりません。
システムにLLMを組み込みたい人にとっては大問題ですよね。
マイクロソフトが発表したTypeChatを使えば、この問題を解決できます。
この記事ではTypeChatのインストールと使い方を解説します。
TypeChatとは?
TypeChatは、LLMからの応答を指定した型で受け取るためのツールです。
TypeScriptで定義した型に従って応答を受け取ることができます。
TypeChat自体もTypeScriptを使って開発されています。
TypeChatのインストール
TypeChatはnpmでインストールできますが(npm install typechat
)、
ここではTypeChatのリポジトリに含まれるExamplesを試すため、以下のようにソースからインストールします。
git clone https://github.com/microsoft/TypeChat
cd TypeChat
npm install
インストールできたら、次のコマンドでビルドします。
npm run build-all
OpenAIのAPIキーの設定
続いてOpenAIのAPIキーを設定します。
先ほどcloneしたTypeChatのディレクトリ直下に.envファイルを作成します(TypeChat/.env)。
.envファイルには以下のように記述します。
APIキーはご自身で用意したものを設定してください(APIキーの取得方法はこちら)。
OPENAI_MODEL=gpt-3.5-turbo # gpt-4
OPENAI_API_KEY=[OpenAIのAPIキー]
これで設定は完了です。
TypeChatのサンプルを試す
いよいよTypeChatを試します。
ここでは公式で用意されているExamplesを使ってTypeChatの動作を見ていきます。
以下のページでTypeChatのExamplesの一覧と説明を見ることができます。
まずは「Sentiment」から見てみましょう。
「これがTypeChatのHello, World」と書かれています。
Sentimentはユーザーの入力したテキストの感情を「positive」「negative」「neutral」のいずれかに分類します。
通常、GPTに「このテキストの感情は?」という質問をすると回答が長い文章になったり、余計な情報が付加されたりして回答の型を制限できません。
しかし、TypeChatを使えば、以下の型にしたがって「positive」「negative」「neutral」で返してくれるようになります。
export interface SentimentResponse {
sentiment: "negative" | "neutral" | "positive"; // The sentiment of the text
}
さっそくSentimentのサンプルを実行してみましょう。
TypeChatのディレクトリ直下で以下のコマンドを実行します。
node ./examples/sentiment/dist/main.js ./examples/sentiment/dist/input.txt
すると、次のようにテキストとそこに込められた感情(neutral, positive, negative)が対になって表示されます。
😀> hello, world
The sentiment is neutral
😀> TypeChat is awesome!
The sentiment is positive
😀> I'm having a good day
The sentiment is positive
😀> it's very rainy outside
The sentiment is negative

すべてのテキストに対して同じ形式でGPTの応答を受け取ることができています。
このように事前に用意されたテキストではうまく動作していますが、こちらで用意したテキストでもうまくいくか試してみましょう。
上記のテキストは以下のファイルに記述されています。ここに任意のテキストを追記します。
TypeChat/examples/sentiment/dist/input.txt
次のように日本語のテキストを入れてみましょう。
hello, world
TypeChat is awesome!
I'm having a good day
it's very rainy outside
こんにちは!
素晴らしい天気ですね!
雨なのでつらいです
悲しいこともあるけどがんばりましょう
書き換えたら一度以下のコマンドを実行して変更をビルドします。
npm run build-all
ビルドできたらもう一度、以下のコマンドを実行しましょう。
node ./examples/sentiment/dist/main.js ./examples/sentiment/dist/input.txt
すると、以下のように日本語で追記したテキストも同じ形式で感情を分類できています。
😀> hello, world
The sentiment is neutral
😀> TypeChat is awesome!
The sentiment is positive
😀> I'm having a good day
The sentiment is positive
😀> it's very rainy outside
The sentiment is negative
😀> こんにちは!
The sentiment is neutral
😀> 素晴らしい天気ですね!
The sentiment is positive
😀> 雨なのでつらいです
The sentiment is negative
😀> 悲しいこともあるけどがんばりましょう
The sentiment is positive
日本語で入力してもまったく回答のフォーマットが変わっていません。
素晴らしいですね。
TypeChatはどうやって型をそろえているか?
TypeChatはどのようにして応答の型をそろえているのでしょうか?
実は結構かんたんな仕組みです。
まず、開発者が求める応答の型を、次のようにTypeScriptで記述したとします。
export interface SentimentResponse {
sentiment: "negative" | "neutral" | "positive"; // The sentiment of the text
}
するとTypeChatは次のようなプロンプトを作成します。
`You are a service that translates user requests into JSON objects of type "${validator.typeName}" according to the following TypeScript definitions:\n` +
`\`\`\`\n${validator.schema}\`\`\`\n` +
`The following is a user request:\n` +
`"""\n${request}\n"""\n` +
`The following is the user request translated into a JSON object with 2 spaces of indentation and no properties with the value undefined:\n`;
上述のSentimentの例では、validator.typeNameに「SentimentResponse」を、validator.schemaに「TypeScriptで定義した型」を、requestに分析させるテキストを入れてプロンプトを作っています。
これは次のように型を明示してChatGPTに指示を出すのと同じです。
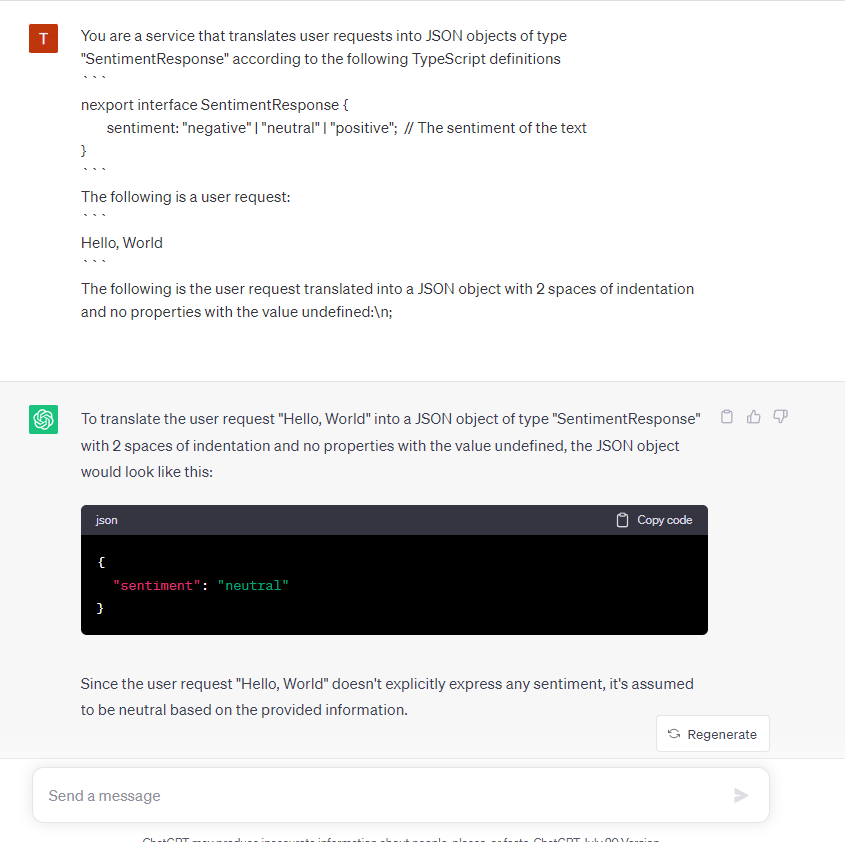
この応答からJSON部分を切り出しています。
ただし、これだけだと指定した通りの型にならない場合もあります。
そこでTypeChatでは回答が指定した型かを検証して、そうでない場合に以下のような修正プロンプトを送信して形式を修正させています。
`The JSON object is invalid for the following reason:\n` +
`"""\n${validationError}\n"""\n` +
`The following is a revised JSON object:\n`;
これを正しい応答になるまで繰り返すというわけです。
まとめ
この記事では、LLMからの応答を構造化された形式に保つTypeChatを解説しました。
TypeChatを使えば応答の型が狙った通りになり、LLMをシステムに組み込みやすくなります。
簡単に使えるのでぜひ使ってみてください。
記事の内容にわからないことがある方は以下のアカウントに気軽にDMしてください!
また、最新の技術の活用方法など役に立つツイートを心がけているので、ぜひフォローしてもらえると嬉しいです!